Inside look of Machine learning success stories
- The International Data Corporation (IDC) estimates that spending on machine learning will reach $77.6 billion by 2022. Researchers estimate that machine learning has potential to add $2.6 trillion in value to the marketing and sales industry by 2020.
- According to Forbes, patents of machine learning grew at compounded annual growth rate (CAGR) 34% in the period 2013-2018.
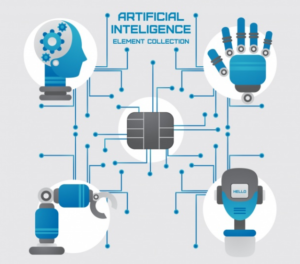
Machine learning is among the most significant technological development in recent time period. Success of machine learning in many areas are now evolutionary rather that revolutionary. Some few amazing stories are include cars are self driving, accurate Xray & Pathology predictions with Neural Networks,
NetFlix recommendations and Speech recognition that actually understands what we say almost perfectly, for example Siri and Ok Google seem to work amazingly well. In future, as times goes by, the level of accuracy will rise, such as flying cars will be possible.
Want to know about some real examples of machine learning that are currently in action? Here are some famous platforms and top companies that are using the power of machine learning in new and exciting ways and also a peek into the future of machine learning.
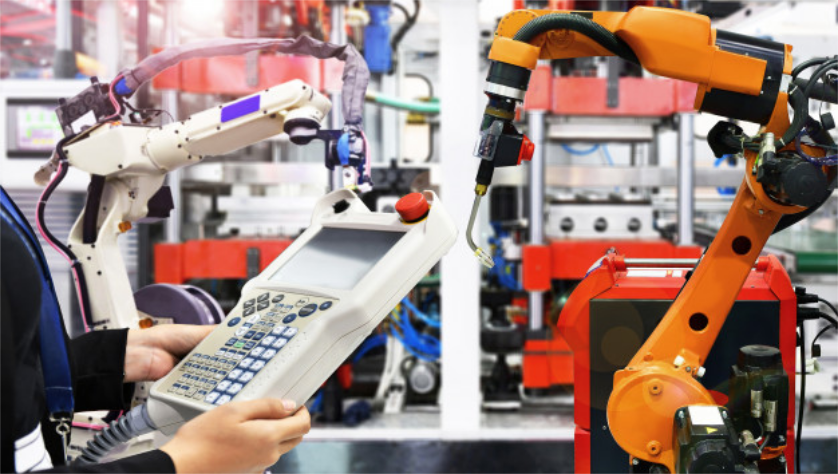
Pinterest: A platform for Augmented Content Discovery
Machine learning today, touches virtually each and every aspect of Pinterest’s business operations, ether it’s from spam moderation and content creation to monetization of advertisement and reducing toss of email newsletter subscribers.
Google: Machine that dreams?
Neural networks have diversified into such fields as anti-aging technology, medical devices, and most importantly exciting for tech nerds The most recognized developments in Google’s neural network research has been the “machine that dreams” which is basically a Deep Mind network, It’s the similar network that produced . They’re not just recognizing images, they’re creating them.
Is this tech really showing us the dreams of a machine? So, first of all, it starts by feeding it a photo or some other image. The neural network looks for familiar patterns in the image and enhances those patterns. Then it repeats the process with the same image, which creates a feedback loop.
For example, if a cloud looks a little bit like a bird, the network will make it look more like a bird as said by Google in a Google AI blog, when it first unveiled this project. This will eventually make the network recognize the bird even more strongly on the next pass until a highly comprehensive and accurate bird appears out of nowhere.
As said by Steven Hansen, an intern at Google’s Deep Mind AI lab in London, the end result is fascinating as well as little disturbing. If you feed a photo of yourself into the neural net and it finds something that kind of looks like a dog in the lines of your faces, it turns that part of your face into a dog. “It’s almost similar as if the neural net is hallucinating,” “It sees dogs everywhere” Or, if you feed the neural net an image of random noise, it may produce a tree or a tower or a whole city of towers. In that same noise, it might find the faint images of a pig and a snail, creating a rather frightening new creature by combining the two. Think: machines on LSD.
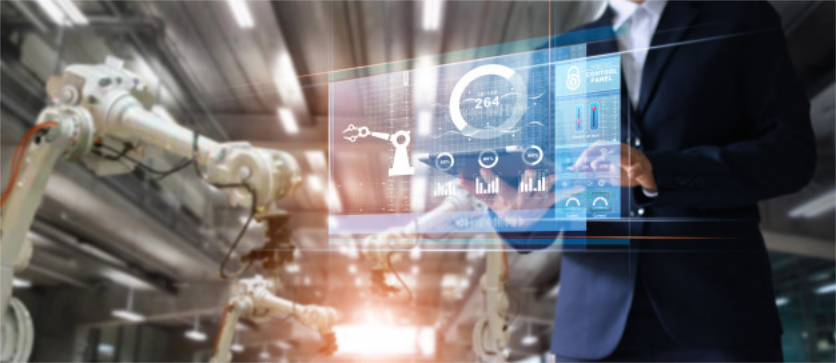
Uber: Using machine learning to modify transportation?
Uber is undoubtedly the biggest cab service provider worldworld that has been leveraging futuristic technologies for optimizing processes and enhancing better customer service. Uber engineering is constantly exploring various methods in order to provide better services for maintaining the lead in market share.
Uber Engineering is always working on machine learning (ML) and other advanced technologies to use it even more precisely to serve their customers better. Uber is using Machine learning to enable an effective ride-sharing marketplace.
Suspicious or fraudulent accounts along with the suggestion for convenient pickup and dropoff points are generally identified by Machine learning . Uber also uses MI to optimize their maps, right from the destination search and techniques such as long short-term memory (LSTM) networks that help predict the future of the Marketplace and the occurrence of extreme events even before happening.
Uber adopts a platform called ML-enabled Natural Language Processing (NLP) that generates actionable responses for customer support tickets, chatbots to make driver onboarding easier, and suggested in-app replies. Along with the commitment to driver partners, Uber has been using NLP platform with deep learning process to optimize the recommended actions. Uber also uses the system to alert drivers of the regions with future demand and it makes sure that there are always enough cabs present in the predicted areas of demand and thus bridges the supply-demand gap. All thanks to machine learning technology of Uber that helps them from losing customers to its competitors.
Overall Machine Learning enables precise coordination, real-time decision making, and learning needed to monitor the all in all movement of the transportation network. Machine learning algorithms enable us to “see into the future” as accurately and clearly as possible across both space and time period also enables us to generate forecasts of supply, demand, and other quantities and variables in real time for up to several weeks ahead.